引用本文: | 陈儒森,吉小超,张梦清,于鹤龙,尹艳丽,周俊,魏敏,史佩京,张伟.机器学习在激光熔覆涂层缺陷检测中的研究现状与进展[J].中国表面工程,2024,37(5):112~137 |
| CHEN Rusen,JI Xiaochao,ZHANG Mengqing,YU Helong,YIN Yanli,ZHOU Jun,WEI Min,SHI Peijing,ZHANG Wei.Progress and Research Status in Machine Learning for Defect Detection in Laser Cladding Coatings[J].China Surface Engineering,2024,37(5):112~137 |
|
|
|
本文已被:浏览 720次 下载 465次 |
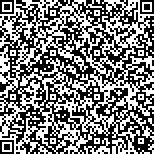 码上扫一扫! |
|
机器学习在激光熔覆涂层缺陷检测中的研究现状与进展 |
陈儒森1,吉小超1,张梦清2,于鹤龙3,尹艳丽3,周俊4,魏敏1,史佩京1,张伟1
|
1.佛山大学机电工程与自动化学院 佛山 528225 ;2.军事科学院防化研究院 北京 102205 ;3.陆军装甲兵学院装备再制造技术国防科技重点实验室 北京 100072 ;4.成都国营锦江机器厂 成都 610043
|
|
摘要: |
机器学习作为人工智能领域的核心分支,通过算法分析数据,从中发现规律和模式,进而做出预测和决策,近年来在激光熔覆领域得到广泛应用。激光熔覆过程中形成的各类缺陷严重影响熔覆层的质量与性能,熔覆质量的可靠性与可重复性是激光熔覆技术面临的最大挑战。数据驱动的机器学习算法可用于激光熔覆过程监测与缺陷检测、反馈调控熔覆工艺、优化抑制熔覆缺陷,已成为本领域的研究热点。综述激光熔覆过程中产生的缺陷类型与成形机制,概述激光熔覆过程中产生的信号特征及其监测原理与手段,总结机器学习方法在激光熔覆过程中信号特征提取、缺陷分类识别与预测的研究进展,归纳缺陷检测的典型机器学习模型与算法。结果表明,机器学习算法可有效用于激光熔覆涂层缺陷检测,构建特征信号与涂层缺陷及熔覆工艺间的关系。目前研究采用的机器学习算法以监督学习算法为主,无监督和半监督学习算法对数据标注要求低,已在激光熔覆过程监测领域获得关注,并展现出巨大的潜力。研究结果为机器学习方法在激光熔覆领域的研究指出了热点与方向。 |
关键词: 激光熔覆 机器学习 缺陷检测 人工智能 |
DOI:10.11933/j.issn.1007-9289.20231115002 |
分类号:V261;TG665 |
基金项目:季华实验室基金(X220971UZ230);国家自然科学基金(52075544);广东省基础与应用基础研究基金(2022A1515110649) |
|
Progress and Research Status in Machine Learning for Defect Detection in Laser Cladding Coatings |
CHEN Rusen1,JI Xiaochao1,ZHANG Mengqing2,YU Helong3,YIN Yanli3,ZHOU Jun4,WEI Min1,SHI Peijing1,ZHANG Wei1
|
1.School of Mechatronic Engineering and Automation, Foshan University, Foshan 528225 , China ;2.Research Institute of Chemical Defense, Academy of Military Sciences, Beijing 102205 , China ;3.National Key Laboratory for Remanufacturing, Army Academy of Armored Forces, Beijing 100072 , China ;4.Chengdu State-owned Jinjiang Machine Factory, Chengdu 610043 , China
|
Abstract: |
The rapid development of artificial intelligence technology has led to significant changes and opportunities across various sectors. Machine learning, an important branch of artificial intelligence, can discover laws and patterns from data to make predictions and decisions. Furthermore, it has been widely used in the field of laser cladding in recent years. Laser cladding technology has emerged as a transformative method with numerous advantages, positioning it as a key player in various industrial applications. Its advantages, including high fusion efficiency, optimal material utilization, robust bonding, and extensive design flexibility, render it indispensable for repairing complex surface defects in metal parts. The occurrence of defects during the cladding process can significantly affect the quality and performance of the cladding layer. Ensuring the reliability and repeatability of cladding quality remains a significant challenge in the field of laser cladding technology. In this study, the application of machine learning algorithms in the field of laser cladding defect assessment is explored. A comprehensive and in-depth analysis of common defects and their formation mechanisms in the laser cladding process is provided. The acoustic, optical, and thermal signals generated during the cladding process are summarized, and the corresponding relationships between these signals and the cladding defects are described. Commonly used methods, sensors, and signal characteristics for monitoring the laser cladding process are summarized. Additionally, the classification and features of machine learning algorithms are organized and their use in signal processing is reviewed during the laser cladding process. The classification and characteristics of machine learning algorithms and their applications in laser cladding signal processing are summarized. Machine learning algorithms have been employed in detecting defects in laser cladding, typically by constructing datasets from features extracted from collected signals, the cladding process, and defect characteristics. These algorithms are used to establish relationships between the signals, defects, and the process. However, most current studies on laser cladding monitoring focus on a single pass or a small area of the cladding layer. The use of such small datasets can lead to model overfitting, thereby reducing the accuracy of defect detection. Nevertheless, the application of these algorithms facilitates the introduction of a dynamic feedback control mechanism that optimizes the cladding process and effectively mitigates defects. The convergence of laser cladding and machine learning has emerged as a vibrant area of research, tackling crucial issues and expanding the limits of quality assurance and process optimization. Researchers, both domestically and internationally, have examined pores, cracks, and other defects at various scales through experiments and simulations. However, the mechanisms behind these defects and their impact on the quality of cladding are not yet fully understood. There is a need for more comprehensive methods to study the laser cladding process. Developing a quantitative evaluation system that links the laser cladding process, signal data, and defect quality is a critical challenge in ensuring the reliability of laser cladding quality. Currently, various sensors, including acoustic, optical, and thermal types, are utilized to monitor the laser cladding process. These sensors aid in examining the relationship between the process signals, defects, and quality. However, the limitations in sensor accuracy and the efficiency of defect feature extraction pose challenges in establishing a precise process-signal-defect relationship. The predominant machine learning algorithms used in current research are supervised learning algorithms. However, unsupervised and semi-supervised learning algorithms, which require less data labeling, are drawing attention in the fields of laser melting and cladding process monitoring, demonstrating significant potential. This review emphasizes the current research hotspots and directions for applying machine learning methods in laser cladding. |
Key words: laser cladding machine learning defects detection artificial intelligence |
|
|
|
|